In data science, Frequentist vs. Bayesian statistics is an age-old debate. While the approaches are different, is there a practical or pragmatic difference? This article defines what each approach entails, the individual pros and cons, and what it means to apply them in the real world.
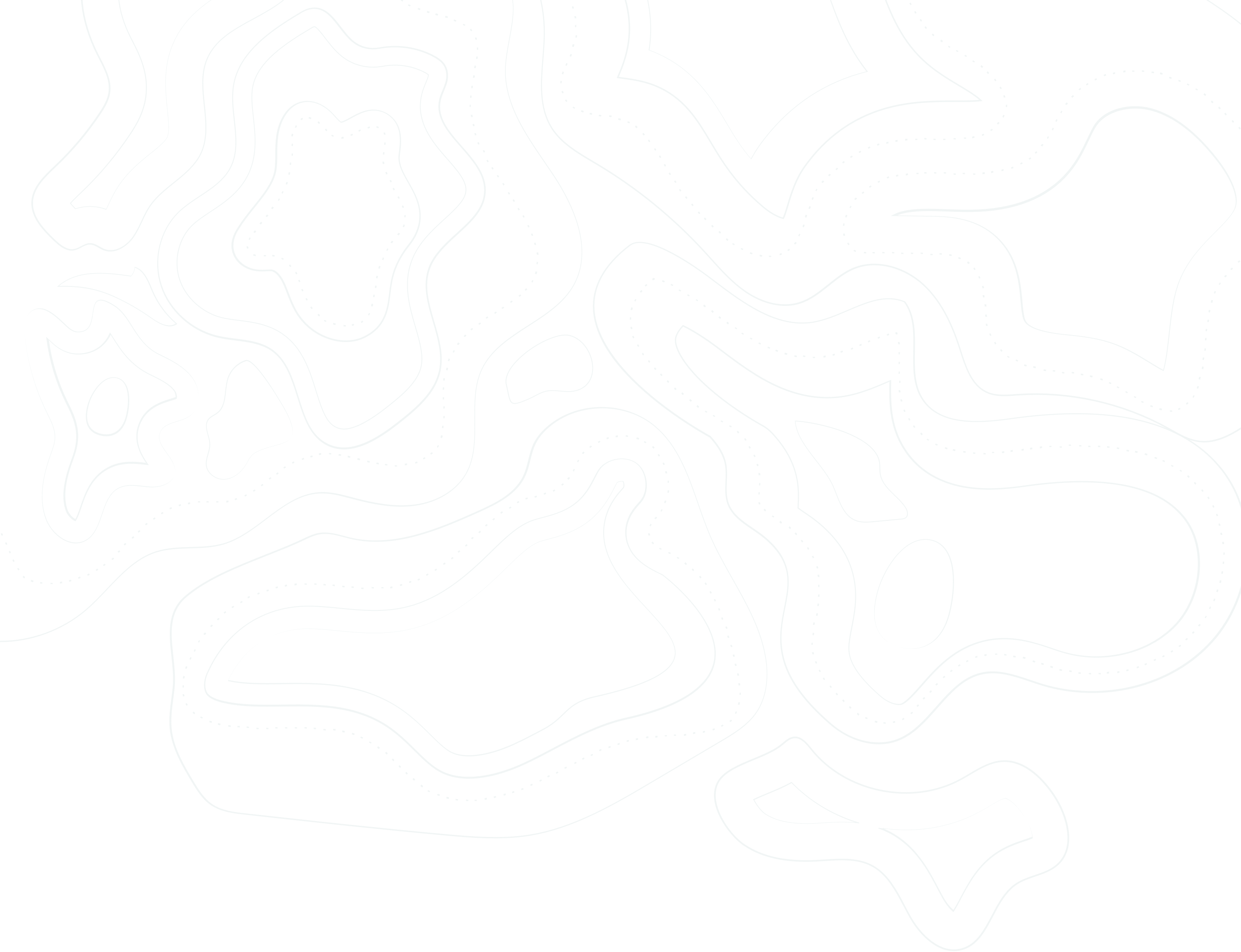
Are you a Frequentist or a Bayesian?
Article May 14, 2022
Suman Sridhar
The Frequentist Approach
As CXL.com says, a frequentist method makes predictions based on the underlying truths of the experiment using only data from the current experiment. It assumes no prior probability, and it's the approach that A/B testing uses the most.
When applying frequentist statistics, you'll come across the term p-value. The p-value is the calculated probability of obtaining an effect at least as extreme as the one in your sample data. A small p-value means that there's a slight chance that your results are entirely random. A larger p-value means that your results are highly likely to be random and not because of what you did in the experiment. The smaller your p-value, the more statistically significant your results are.
The Bayesian Approach
The Bayesian approach assumes prior probability. Use the prior data - the "prior" for shorthand - combined with current experiment data to make a conclusion with the test at hand.
The approach works as follows:
- Define the prior distribution based on your subjective beliefs about a parameter.
- Collect the relevant data.
- Update the prior distribution with the data using Bayes' theorem.
- Analyze the distribution and summarize it----the mean median, standard deviation, and quantiles.
As Boundless Rationality says, "A fundamental aspect of Bayesian inference is updating your beliefs in light of new evidence. Essentially, you start out with a prior belief and then update it in light of new evidence. An important aspect of this prior belief is your degree of confidence in it."
In the Bayesian view, a hypothesis has a probability. In the frequentist view, you test a hypothesis with no associated probability.
The Plusses and Minuses of Both Approaches
One of the big pros of frequentist inferences is that the p-value is objective---all statisticians will agree on it. Then the individual will decide to reject the original, or null, hypothesis.
Secondly, it requires establishing control to reduce experimenter bias. Thirdly, it has been used for over a century.
Most of us learn frequentist statistics in entry-level courses. Frequentist arguments are more counter-factual and resemble lawyers' type of logic in court. For example, a t-test, where we ask, 'Is this variation different from the control?' is a fundamental building block of this approach.
Naturally, the frequentist method has its critics. One complaint is that it's ad-hoc and doesn't require deductive logic. Another is that you need to specify experiments ahead of time, which may create paradoxical results.
Experts praise the Bayesian method because you can communicate results based on the probability of a hypothesis. Other merits are:
- You use data as it comes in. You don't have to specify experiments ahead of time.
- The prior may be subjective, but you can specify the assumptions used to arrive at it. Other people can challenge it or try different priors.
- You can try out different priors to gauge how sensitive your results are.
- It's logically rigorous.
The Bayesian approach doesn't give you a correct way to choose a prior. A disadvantage is the subjectivity of a prior. How trustworthy and solid will your conclusions be?
Applying Frequentist/Bayesian
As Towards Data Science says, Frequentist statistics is about repeatability and gathering data. It's harder to apply it in situations with no repeatable data. For example, if you flip a coin and the probability of it landing heads is 0.5, you would see heads 50 percent of the time.
Some data scientists say Bayesian is easier to understand and compute. For example, you can determine a patient's probability of contracting a liver disease if they're an alcoholic. The frequentist definition of probability is more binary - the patient either has the disease or not.
Frequentist measures like p-value and confidence research are prominent in research, particularly in the life sciences. However, according to MIT, Bayesian methods are more often used in machine learning and genetics fields. Several large, ongoing clinical trials use Bayesian protocols.
Ultimately, it's hard to declare that one approach is better than the other. Both approaches are equally impacted by variance, though the Bayesian approach works better on population data. While it seems like the Bayesian method is more popular, you always have to factor in the subjectivity of the prior. Top statisticians say that the most effective approaches to complex problems often draw on the best insight from both methods.
Conclusion
Both the Frequentist and Bayesian approaches work better in different situations. There are even hybrid techniques that employ the best of both worlds. Data scientists will still use both methods to make and prove hypotheses or assess the probability of an event for decades to come.
Looking for a guide on your journey?
Ready to explore how human-machine teaming can help to solve your complex problems? Let's talk. We're excited to hear your ideas and see where we can assist.
Let's Talk