There has been a lot of hype over the past couple of years about Artificial Intelligence and Machine Learning, and many organizations are just now in a position to consider an AI/ML project. Thoughtful review of five aspects of your organization can help you decide to take the leap into an AI/ML project.
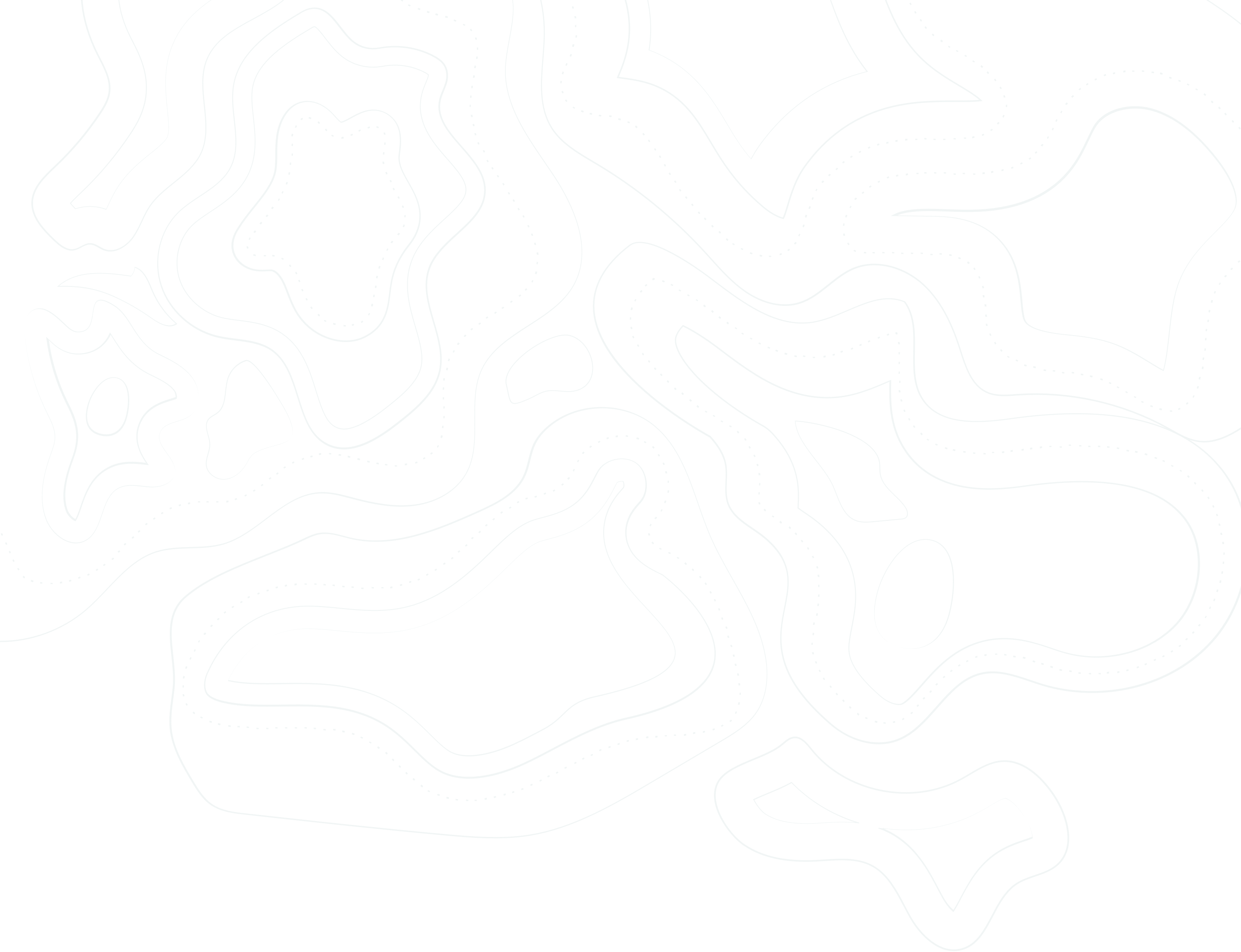
Are You Ready for Your First AI/ML Project?
Article Jan 11, 2020
Pomiet
While there has been a lot of hype for a couple of years about Artificial Intelligence and Machine Learning, many organizations are just now in a position to consider an AI/ML project. At POMIET we like to approach machine learning projects as the development of a synergistic system between man and machine. Machine learning in practice requires human application of the scientific method and human communication skills. The scientific method is not fool proof and may lead to dead ends. However, it will also facilitate learning and minimize the costs of false starts. Successful organizations have the proper data, infrastructure, expertise, and close collaboration between analytics and business subject matter experts to follow good processes and attain solid ROI.
Thoughtful review of five aspects of your organization can help you decide to take the leap into an AI/ML project. These aspects are the state of your data, your available resources, subject matter experts, tools and expertise, and your users.
State of Your Data: AI/ML solutions are highly dependent on the available data for training and verification purposes. Just because you have a lot of data does not mean it is ready for consumption by AI/ML algorithms. Data Engineers may need to spend weeks or months wrangling your data in preparation for an AI/ML approach. Simulated data may be appropriate under certain experimental conditions, but it is not appropriate for production systems.
Available Resources: Some AI/ML approaches are computationally expensive. Be sure that you have budgeted for this expense, or have access to the powerful machines that will handle the load.
Subject Matter Expert: You may have heard horror stories of Machine Learning systems that have been deployed with strong bias due to the training data used in the system development. While a subject matter expert will bring blinders and bias to the data, it is likely that she will be more aware of the bias than the data scientists who are building the machine learning models. Due to the strong dependence on the data, and proper interpretation of the data, algorithm development must be supported by a knowledgable, detail-focused SME.
Tools and Expertise: Not all tools are created equal. Not all statisticians, computer scientists, or data scientists are created equal. With this in mind, be sure to be aware of the areas of excellence and limitation of both the tools and the people building the models.
Users: For synergistic partnership between human and machine to be developed, the users must be involved throughout the development and evaluation of the system. If a user receives an unexpected response from a machine, the surprise can lead to positive or negative emotion depending on the level of trust that the user has in the system. Building trust is vital for user adoption of your system.
Review of your potential AI/ML project from these five perspectives will guide you to more work that may need to be done before you start, or give you confidence to proceed. Regardless, be sure to start small and follow the scientific method. This will minimize the risk inherent in AI/ML projects and maximize your ROI.
Looking for a guide on your journey?
Ready to explore how human-machine teaming can help to solve your complex problems? Let's talk. We're excited to hear your ideas and see where we can assist.
Let's Talk